A huge number of informal messages are posted every day in social network sites, blogs and discussion forums. Emotions seem to be frequently important in these texts for expressing friendship, showing social support or as part of online arguments. Algorithms to identify sentiment and sentiment strength are needed to help understand the role of emotion in this informal communication and also to identify inappropriate or anomalous affective utterances, potentially associated with threatening behaviour to the self or others. Nevertheless, existing sentiment detection algorithms tend to be commercially-oriented, designed to identify opinions about products rather than user behaviours. This article partly fills this gap with a new algorithm, SentiStrength, to extract sentiment strength from informal English text, using new methods to exploit the de-facto grammars and spelling styles of cyberspace. Applied to MySpace comments and with a lookup table of term sentiment strengths optimised by machine learning, SentiStrength is able to predict positive emotion with 60.6% accuracy and negative emotion with 72.8% accuracy, both based upon strength scales of 1-5. The former, but not the latter, is better than baseline and a wide range of general machine learning approaches.
每天在社交网站、博客和论坛上都会发布大量非正式消息。 在这些文本中,情感似乎对于表达友谊、显示社会支持或作为在线争论的一部分很重要。 需要识别情绪和情绪强度的算法来帮助理解情绪在这种非正式交流中的作用,并识别不恰当或异常的情感表达,这些表达可能与对自己或他人的威胁行为有关。 尽管如此,现有的情绪检测算法往往是面向商业的,旨在识别对产品的看法而不是用户行为。 本文使用新算法 SentiStrength 部分填补了这一空白,该算法使用新方法从非正式英语文本中提取情感强度,利用网络空间的实际语法和拼写风格。 应用于 MySpace 评论和通过机器学习优化的术语情绪强度查找表,SentiStrength 能够以 60.6% 的准确度预测积极情绪,以 72.8% 的准确度预测消极情绪,两者均基于 1-5 的强度等级。 前者,而不是后者,优于基线和广泛的通用机器学习方法。
Introduction
Most opinion mining algorithms attempt to identify the polarity of sentiment in text: positive, negative or neutral. Whilst for many applications this is sufficient, texts often contain a mix of positive and negative sentiment and for some applications it is necessary to detect both simultaneously and also to detect the strength of sentiment expressed. For instance, programs to monitor sentiment in online communication, perhaps designed to identify and intervene when inappropriate emotions are used or to identify at-risk users (e.g., Huang, Goh, & Liew, 2007), would need to be sensitive to the strength of sentiment expressed and whether participants were appropriately balancing positive and negative sentiment. In addition, basic research to understand the role of emotion in online communication (e.g., Derks, Fischer, & Bos, 2008; e.g., Hancock, Gee, Ciaccio, & Lin, 2008; Nardi, 2005) would also benefit from fine-grained sentiment detection, as would the growing body of psychology and other social science research into the role of sentiment in various types of discussion or general discourse (Balahur, Kozareva, & Montoyo, 2009; Pennebaker, Mehl, & Niederhoffer, 2003; Short & Palmer, 2008).
大多数意见挖掘算法都试图识别文本中情绪的极性:正面、负面或中性。 虽然对于许多应用程序来说这已经足够了,但文本通常包含正面和负面情绪的混合,对于某些应用程序,有必要同时检测两者并检测表达的情绪强度。 例如,监控在线交流情绪的程序可能旨在识别和干预何时使用不当情绪或识别处于风险中的用户(例如,Huang、Goh 和 Liew,2007 年),需要对强度敏感 表达的情绪以及参与者是否适当地平衡了正面和负面情绪。 此外,了解情绪在在线交流中的作用的基础研究(例如,Derks、Fischer 和 Bos,2008 年;例如,Hancock、Gee、Ciaccio 和 Lin,2008 年;Nardi,2005 年)也将受益于细粒度的 情绪检测,以及越来越多的心理学和其他社会科学研究,研究情绪在各种类型的讨论或一般话语中的作用(Balahur、Kozareva 和 Montoyo,2009 年;Pennebaker、Mehl 和 Niederhoffer,2003 年;Short 和 Palmer , 2008).
A complicating factor for online sentiment detection is that there are many electronic communications media in which text based communication in English seems to frequently ignore the rules of grammar and spelling. Perhaps most famous is mobile phone text language with its abbreviations, emoticons and truncated sentences (Grinter & Eldridge, 2003; Thurlow, 2003) but similar styles are evident in many other forms of computer mediated communication, including chatrooms, bulletin boards and social network sites (Baron, 2003; Crystal, 2006). Widely recognised innovations include emoticons like :-) that are reasonably effective in conveying emotion (Derks, Bos, & von Grumbkow, 2008; Fullwood & Martino, 2007) and word abbreviations like m8 (mate) and u (you) (Thurlow, 2003). Although sometimes seen as poor language use, these are a natural response to the technological affordances and social factors associated with a system (Baron, 2003; Walther & Parks, 2002). These variations cause problems because typical linguistic sentiment analysis programs start with part of speech tagging (e.g., Brill, 1992), which is reliant upon standard spelling and grammar, and/or apply rules that assume at least correct spelling, if not correct grammar. Spelling correction can be useful in this context, but this is based upon the assumption that spelling deviations are likely to be accidental mistakes (Kukich, 1992; Pollock & Zamora, 1984) and so current algorithms are unlikely to work well with deliberately non-standard spellings. Nevertheless, there is a range of common abbreviations and new words that a linguistic algorithm could, in principle, detect. Non-linguistic machine learning algorithms typically predict sentiment based upon occurrences of individual words, word pairs and word triples in documents. These may also perform poorly on informal text because of spelling problems and creativity in sentiment expression, even if a large training corpus is available (see below).
在线情绪检测的一个复杂因素是,有许多电子通信媒体,其中基于英语文本的通信似乎经常忽略语法和拼写规则。 也许最著名的是带有缩写、表情符号和截断句子的手机文本语言(Grinter & Eldridge,2003 年;Thurlow,2003 年),但类似的风格在许多其他形式的以计算机为媒介的交流中也很明显,包括聊天室、公告板和社交网站 (男爵,2003 年;水晶,2006 年)。 广泛认可的创新包括表情符号,如 :-),它们在传达情感方面相当有效(Derks、Bos 和 von Grumbkow,2008 年;Fullwood 和 Martino,2007 年)和单词缩写,如 m8(伴侣)和 u(你)(Thurlow,2003 年) ). 尽管有时被视为语言使用不当,但这是对与系统相关的技术能力和社会因素的自然反应(Baron,2003 年;Walther & Parks,2002 年)。 这些变化会导致问题,因为典型的语言情感分析程序从词性标记开始(例如,Brill,1992),它依赖于标准拼写和语法,和/或应用假定至少正确拼写(如果不是正确语法)的规则。 拼写校正在这种情况下很有用,但这是基于拼写偏差很可能是偶然错误的假设(Kukich,1992 年;Pollock 和 Zamora,1984 年),因此当前的算法不太可能很好地处理故意非标准的问题 拼写。 尽管如此,语言算法原则上可以检测到一系列常见的缩写词和新词。 非语言机器学习算法通常根据文档中单个单词、单词对和单词三元组的出现来预测情绪。 由于拼写问题和情绪表达的创造力,即使有大量的训练语料库可用,这些也可能在非正式文本上表现不佳(见下文)。
The social network site MySpace, the source of the data used in the current study, is known for its young members, its musical orientation and its informal communication patterns (boyd, 2008; boyd, 2008). Probably as a result of these factors 95% of English public comments exchanged between friends contain at least one abbreviation from standard English (Thelwall, 2009). Common features include emoticons, texting-style abbreviations and the use of repeated letters or punctuation for emphasis (e.g., a loooong time, Hi!!!). Comments are typically short (mean 18.7 words, median 13 words, 68 characters) (Thelwall, 2009) but positive emotion is common (Thelwall, Wilkinson, & Uppal, 2010).
社交网站 MySpace 是当前研究中使用的数据来源,以其年轻成员、音乐取向和非正式交流模式而闻名(boyd,2008 年;boyd,2008 年)。 可能由于这些因素,95% 好友之间交换的英语公共评论包含至少一个标准英语的缩写 (Thelwall, 2009)。 常见特征包括表情符号、短信式缩写和使用重复字母或标点符号来强调(例如,很长的时间,嗨!!!)。 评论通常很短(平均 18.7 个单词,中位数 13 个单词,68 个字符)(Thelwall,2009)但积极情绪很常见(Thelwall、Wilkinson 和 Uppal,2010)。
This article proposes a new algorithm, SentiStrength, which employs several novel methods to simultaneously extract positive and negative sentiment strength from short informal electronic text. SentiStrength uses a dictionary of sentiment words with associated strength measures and exploits a range of recognised non-standard spellings and other common textual methods of expressing sentiment. SentiStrength was developed through an initial set of 2,600 human-classified MySpace comments, and evaluated on a further random sample of 1,041 MySpace comments. Note that in some articles, but not in emotion psychology, the term sentiment refers to affect split into positive, negative and neutral whereas the term emotion refers to more differentiated affect (e.g., happy, sad, frightened). In contrast, the two terms are used as synonyms here, with their meaning effectively defined by the coder instructions described below. The main novel contributions of this paper are: a machine learning approach to optimise sentiment term weightings; methods for extracting sentiment from repeated letter non-standard spelling in informal text; and a related spelling correction method. In addition, the paper introduces a dual 5-point system for positive and negative sentiment, a corpus of 1,041 MySpace comments for this system, and a new overall sentiment strength detection system that combines novel and existing methods.
本文提出了一种新算法 SentiStrength,它采用多种新颖的方法从简短的非正式电子文本中同时提取正面和负面情绪强度。 SentiStrength 使用带有相关强度度量的情感词词典,并利用一系列公认的非标准拼写和其他表达情感的常见文本方法。 SentiStrength 是通过一组初始的 2,600 条人工分类的 MySpace 评论开发的,并根据 1,041 条 MySpace 评论的进一步随机样本进行了评估。 请注意,在一些文章中,但不是在情绪心理学中,sentiment 一词指的是分为积极、消极和中性的情感,而 emotion 一词指的是更分化的情感(例如,快乐、悲伤、害怕)。 相反,这两个术语在这里用作同义词,其含义由下面描述的编码器指令有效定义。 本文的主要创新贡献是:一种优化情感术语权重的机器学习方法; 从非正式文本中的重复字母非标准拼写中提取情感的方法; 以及相关的拼写纠正方法。 此外,本文还介绍了一个正面和负面情绪的双 5 分系统,该系统包含 1,041 条 MySpace 评论的语料库,以及一个结合了新颖和现有方法的新的整体情绪强度检测系统。
Background and Related Work
This literature review section discussed related opinion mining/sentiment analysis research as well as some relevant contributions from emotion psychology.
本文献综述部分讨论了相关的意见挖掘/情感分析研究以及情绪心理学的一些相关贡献。
Opinion mining
Opinion mining, also known as sentiment analysis, is the extraction of positive or negative opinions from (unstructured) text (Pang & Lee, 2008). The many applications of opinion mining include detecting movie popularity from multiple online reviews and diagnosing which parts of a vehicle are liked or disliked by owners through their comments in a dedicated site or forum. There are also applications unrelated to marketing, such as differentiating between emotional and informative social media content (Denecke & Nejdl, 2009).
意见挖掘,也称为情感分析,是从(非结构化)文本中提取正面或负面意见(Pang & Lee,2008)。 意见挖掘的许多应用包括从多个在线评论中检测电影的受欢迎程度,以及通过车主在专门站点或论坛中的评论来诊断车主喜欢或不喜欢车辆的哪些部分。 还有一些与营销无关的应用程序,例如区分情感和信息社交媒体内容(Denecke & Nejdl,2009)。
Opinion mining typically occurs in two or three stages, although more may be needed for some tasks (e.g., Balahur et al., 2010). First, the input text is split into sections, such as sentences, and each section tested to see if it contains any sentiment: if it is subjective or objective (Pang & Lee, 2004). Second, the subjective sentences are analysed to detect their sentiment polarity. Finally, the object about which the opinion is expressed may be extracted (e.g., Gamon, Aue, Corston-Oliver, & Ringger, 2005). Opinion mining normally deals with only positive and negative sentiment rather than discrete emotions (e.g., happiness, surprise), does not detect sentiment strength (but sometimes uses the strength of association of words with positive or negative sentiment, e.g., Kaji & Kitsuregawa, 2007), and does not simultaneously identify both positive and negative emotions. Nevertheless, such opinion mining research can aid the simultaneous assessment of positive and negative sentiment strength both because of its general insights into sentiment analysis and also because most techniques could, in theory, be repurposed for this new task. For example, phrase analysis techniques could be applied to identify both positive and negative sentiment even within individual sentences (Choi & Cardie, 2008; Wilson, 2008; Wilson, Wiebe, & Hoffman, 2009).
意见挖掘通常分两个或三个阶段进行,尽管某些任务可能需要更多阶段(例如,Balahur 等人,2010 年)。 首先,输入文本被分成几个部分,比如句子,每个部分都经过测试,看它是否包含任何情感:是主观的还是客观的(Pang & Lee,2004)。 其次,分析主观句子以检测其情感极性。 最后,可以提取表达意见的对象(例如,Gamon、Aue、Corston-Oliver 和 Ringger,2005 年)。 意见挖掘通常只处理积极和消极的情绪,而不是离散的情绪(例如快乐、惊讶),不检测情绪强度(但有时会使用单词与积极或消极情绪的关联强度,例如,Kaji & Kitsuregawa,2007 ), 并且不会同时识别积极和消极情绪。 尽管如此,这种观点挖掘研究可以帮助同时评估正面和负面情绪强度,因为它对情绪分析有一般见解,而且从理论上讲,大多数技术都可以重新用于这项新任务。 例如,即使在单个句子中,短语分析技术也可用于识别正面和负面情绪(Choi & Cardie, 2008; Wilson, 2008; Wilson, Wiebe, & Hoffman, 2009)。
Opinion mining algorithms often use machine learning to identify general features associated with positive and negative sentiment, where these features could be a subset of the words in the document, parts of speech or n-grams (i.e., the frequency of occurrence of all n consecutive words, where n is typically 1, 2, or 3) (Abbasi, Chen, Thoms, & Fu, 2008; Ng, Dasgupta, & Arifin, 2006; Tang, Tan, & Cheng, 2009). Other features used with some success include: emoticons in online movie reviews (Read, 2005), which seem so be more domain-independent than words; lexico-syntactic patterns (e.g., Riloff & Wiebe, 2003); and artificial features derived from adjective polarity lists (Ng et al., 2006). The additional features typically provide small but significant increases in performance. Rules-based methods have also been used to identify structures in sentences associated with sentiment (Prabowo & Thelwall, 2009; Wu, Chuang, & Lin, 2006). Two recurring machine learning issues are feature selection and classification algorithm choice.
意见挖掘算法通常使用机器学习来识别与正面和负面情绪相关的一般特征,其中这些特征可以是文档中单词的子集、词性或 n-gram(即所有 n 个连续的出现频率) 词,其中 n 通常为 1、2 或 3)(Abbasi、Chen、Thoms 和 Fu,2008 年;Ng、Dasgupta 和 Arifin,2006 年;Tang、Tan 和 Cheng,2009 年)。 其他成功使用的功能包括:在线电影评论中的表情符号(Read,2005 年),它似乎比文字更独立于领域; 词汇句法模式(例如,Riloff & Wiebe,2003); 以及来自形容词极性列表的人工特征 (Ng et al., 2006)。 附加功能通常会提供小而显着的性能提升。 基于规则的方法也被用于识别与情感相关的句子结构(Prabowo & Thelwall, 2009; Wu, Chuang, & Lin, 2006)。 两个反复出现的机器学习问题是特征选择和分类算法选择。
Feature selection, data processing to remove the least useful n-grams, has been shown to slightly improve classification performance, for example by choosing a restricted set of features (e.g., 5000) that score highest on a measure like information gain (Riloff, Patwardhan, & Wiebe, 2006), or log likelihood (Gamon, 2004). When using n-grams (and lexico-syntactic patterns) small improvements can also be made by pruning the feature set of features that are subsumed by simpler features that have stronger information gain values (Riloff et al., 2006). For example, if “love” has a much higher information gain value than “I love” then the bigram can be eliminated without much risk of loss of power for the subsequent classification. An entropy-weighted genetic algorithm can also perform better than standard feature reduction approaches (Abbasi, Chen, & Salem, 2008).
特征选择,数据处理以去除最不有用的 n-gram,已被证明可以略微提高分类性能,例如通过选择在信息增益等度量上得分最高的一组受限特征(例如 5000)(Riloff,Patwardhan , & Wiebe, 2006) 或对数似然 (Gamon, 2004)。 当使用 n-gram(和词典句法模式)时,也可以通过修剪由具有更强信息增益值的更简单特征所包含的特征的特征集来进行小的改进(Riloff 等人,2006)。 例如,如果“love”比“I love”具有更高的信息增益值,则可以在不损失后续分类能力的情况下消除二元组。 熵加权遗传算法的性能也优于标准特征缩减方法 (Abbasi, Chen, & Salem, 2008)。
In terms of classification algorithms, support vector machines (SVMs) are widely used (Abbasi et al., 2008; Abbasi et al., 2008; Argamon et al., 2007; Gamon, 2004; Mishne, 2005; Wilson, Wiebe, & Hwa, 2006) because they seem to perform as well or better than other methods in most machine learning contexts. Nevertheless, with a few exceptions (Read, 2005; Wilson et al., 2006), explicit comparisons with other methods have not been included in opinion mining publications.
在分类算法方面,支持向量机 (SVM) 被广泛使用 (Abbasi et al., 2008; Abbasi et al., 2008; Argamon et al., 2007; Gamon, 2004; Mishne, 2005; Wilson, Wiebe, & Hwa, 2006),因为在大多数机器学习环境中,它们的表现似乎与其他方法一样好或更好。 然而,除了少数例外(Read,2005 年;Wilson 等人,2006 年),与其他方法的明确比较尚未包含在意见挖掘出版物中。
Many other approaches have also been used to detect sentiment in text. One is to have a dictionary of positive and negative words (e.g., love, hate), such as that found in General Inquirer (Stone, Dunphy, Smith, & Ogilvie, 1966), WordNet Affect (Strapparava & Valitutti, 2004), SentiWordNet (Baccianella, Esuli, & Sebastiani, 2010; Esuli & Sebastiani, 2006) or Q-WordNet (Agerri & García-Serrano, 2010), and to count how often they occur. Modifications of this approach include the identification of negating terms (Das & Chen, 2001), words that enhance sentiment in other words (e.g., really love, absolutely hate) and overall sentence structures (Turney, 2002). A more sophisticated approach is to identify text features that could potentially be subjective in some contexts and then use contextual information to decide whether they are subjective in each new context (Wiebe, Wilson, Bruce, Bell, & Martin, 2004).
许多其他方法也被用于检测文本中的情绪。 一种是拥有正面和负面词(例如,爱、恨)的字典,例如在 General Inquirer(Stone、Dunphy、Smith 和 Ogilvie,1966 年)、WordNet Affect(Strapparava 和 Valitutti,2004 年)、SentiWordNet 中找到的字典 (Baccianella、Esuli 和 Sebastiani,2010 年;Esuli 和 Sebastiani,2006 年)或 Q-WordNet(Agerri 和 García-Serrano,2010 年),并计算它们出现的频率。 这种方法的修改包括否定词的识别 (Das & Chen, 2001),换句话说增强情绪的词 (例如,really love, absolutely hate) 和整体句子结构 (Turney, 2002)。 一种更复杂的方法是识别在某些上下文中可能具有主观性的文本特征,然后使用上下文信息来确定它们在每个新上下文中是否是主观的(Wiebe、Wilson、Bruce、Bell 和 Martin,2004 年)。
An alternative opinion mining technique has used a primarily linguistic approach: simple rules based upon compositional semantics (information about likely meanings of a word based upon the surrounding text) to detect the polarity of an expression (Choi & Cardie, 2008). This gives good results on phrases in newswire documents that are manually coded as having at least medium level positive or negative sentiment. This approach seems particularly suited to cases where there is a large volume of grammatically correct text from which rules can be learned. Nevertheless, a study of poor grammatical quality texts in online customer feedback showed that linguistic approaches could improve classification slightly when added to bag of words (1-grams) approaches, although aggressive feature reduction had a similar impact to adding linguistic features (Gamon, 2004). The improvement was probably due to the large data set available (40,884 documents with an average of 2.26 sentences each), as has been previously claimed for an analysis of informal text (Mishne, 2005). Another approach used a lexicon of appraisal adjectives (e.g., “sort of”, “very”) together with an orientation lexicon to detect movie review polarity. This did not perform as well as unigrams but the combined performance was better than that of unigrams alone (Argamon et al., 2007). Linguistic features have also been successfully used to extend opinion mining to a multi-aspect variant that is able to detect opinions about different aspects of a topic (Snyder & Barzilay, 2007). A promising future approach is the incorporation of context about the reasons why sentiment is used, such as differentiating between intention, arguments and speculation (Wilson, 2008).
另一种意见挖掘技术主要使用语言学方法:基于组合语义的简单规则(关于基于周围文本的单词可能含义的信息)来检测表达式的极性(Choi 和 Cardie,2008)。 这对手动编码为至少具有中等水平正面或负面情绪的新闻专线文档中的短语给出了良好的结果。 这种方法似乎特别适用于有大量语法正确的文本可以从中学习规则的情况。 然而,对在线客户反馈中语法质量差的文本的研究表明,语言学方法在添加到词袋(1-grams)方法时可以略微改善分类,尽管积极的特征减少与添加语言特征具有相似的影响(Gamon,2004 年) ). 改进可能是由于可用的大数据集(40,884 个文档,每个文档平均有 2.26 个句子),正如之前对非正式文本的分析所声称的那样(Mishne,2005)。 另一种方法使用评价形容词词典(例如,“有点”、“非常”)和方向词典来检测电影评论的极性。 这不如 unigrams 表现好,但综合性能优于单独的 unigrams (Argamon et al., 2007)。 语言特征也已成功用于将意见挖掘扩展到能够检测关于主题不同方面的意见的多方面变体 (Snyder & Barzilay, 2007)。 一个有前途的未来方法是结合使用情绪的原因的上下文,例如区分意图、论点和推测 (Wilson, 2008)。
Detecting multiple emotions
Psychology of emotion research argues that whilst positive and negative sentiment are important dimensions, there are many different widely socially-recognised types of emotion and the strength of emotions (arousal level) can vary (e.g., Cornelius, 1996; Fox, 2008). In the dimensional model of emotion from psychology (Russell, 1979), sentiment can always be fundamentally split into two axes: arousal (low to high) and valence (positive to negative). Whilst this model is useful, other research has shown that positive and negative sentiment can coexist (e.g., Fox, 2008, p. 127) and are relatively independent in many contexts – particularly when sentiment levels are not extreme and over longer time periods (Diener & Emmons, 1984; Huppert & Whittington, 2003; Watson, 1988; Watson, Clark, & Tellegen, 1988) and so it also seems reasonable to conceive sentiment as separately-measureable positive and negative components, as encoded in a popular psychology research instrument (Watson et al., 1988).
情绪研究的心理学认为,虽然积极和消极情绪是重要的维度,但有许多不同的广泛社会认可的情绪类型,情绪强度(唤醒水平)可能会有所不同(例如,Cornelius,1996 年;Fox,2008 年)。 在心理学的情绪维度模型中(Russell,1979),情绪总是可以从根本上分为两个轴:唤醒(从低到高)和价(积极到消极)。 虽然这个模型很有用,但其他研究表明,积极情绪和消极情绪可以共存(例如,Fox,2008 年,第 127 页),并且在许多情况下是相对独立的——尤其是当情绪水平不极端且持续时间较长时(Diener & Emmons, 1984; Huppert & Whittington, 2003; Watson, 1988; Watson, Clark, & Tellegen, 1988) 因此,将情绪视为可单独测量的正面和负面成分似乎也是合理的,正如流行的心理学研究工具中所编码的那样 (沃森等人,1988 年)。
There have been some previous attempts to develop algorithms to detect the strength or prevalence of sentiment or emotion in text, or to differentiate between several types of emotion. The LIWC (Linguistic Inquiry and Word Count, www.liwc.net) software from psychology, for example, uses a list of emotion-bearing words to detect positive and negative emotion in text in addition to three specific emotions of particular use in psychology and psychotherapy: anger, anxiety and sadness. It uses simple word counting, measuring the proportion of words falling within an extensive predefined list (e.g., 408 positive and 499 negative words or word stems). The list includes some words that are associated with emotions but do not describe them. For example ‘lucky’ is a positive keyword and ‘loses’ is a negative keyword. In contrast to the machine learning approaches discussed above, these lists have been compiled and validated using panels of human judges and statistical testing.
之前已经有一些尝试开发算法来检测文本中情感或情感的强度或普遍性,或者区分几种类型的情感。 以心理学的 LIWC(Linguistic Inquiry and Word Count,www.liwc.net)软件为例,除了心理学中特别使用的三种特定情绪外,还使用情绪承载词列表来检测文本中的积极和消极情绪。 心理治疗:愤怒、焦虑和悲伤。 它使用简单的单词计数,测量落在广泛的预定义列表中的单词的比例(例如,408 个正面和 499 个负面单词或词干)。 该列表包括一些与情绪相关但不描述它们的词。 例如,“lucky”是一个肯定关键词,“loses”是一个否定关键词。 与上面讨论的机器学习方法相反,这些列表是使用人类评委和统计测试小组编制和验证的。
LIWC calculates the prevalence of emotion in text, rather than attempting to diagnose a text’s overall emotion or emotion strength. It is most suited to longer documents, for which its statistics would be useful indicators of the tendency for emotion to occur. The program uses word truncation for simplicity (e.g., joy* matches any word starting with joy), rather than stemming or lemmatisation, but does not take into account booster words like “very” or the negating effect of negatives (e.g., *not* happy). LIWC has been used by psychology researchers to investigate the connection between language and psychology (Pennebaker et al., 2003) and also as a practical tool, for example to detect how well people are likely to cope with bereavement based upon their language use (Pennebaker, Mayne, & Francis, 1997). A related emotion detection approach differentiates between happy, unhappy and neutral states based upon words used by students describing their daily lives (Wu et al., 2006). This is similar to the typical positive/negative/neutral objective for opinion mining, however.
LIWC 计算文本中情感的普遍性,而不是试图诊断文本的整体情感或情感强度。 它最适合较长的文档,因为它的统计数据可以作为情绪发生趋势的有用指标。 该程序为简单起见使用单词截断(例如,joy* 匹配任何以 joy 开头的单词),而不是词干提取或词形还原,但没有考虑像“very”这样的助推词或否定的否定效果(例如,*not * 快乐的)。 LIWC 已被心理学研究人员用来研究语言与心理学之间的联系(Pennebaker 等人,2003 年),同时也是一种实用工具,例如,根据他们的语言使用情况来检测人们应对丧亲之痛的能力(Pennebaker ,梅恩和弗朗西斯,1997 年)。 一种相关的情绪检测方法根据学生描述日常生活时使用的词语区分快乐、不快乐和中性状态(Wu 等人,2006 年)。 然而,这类似于意见挖掘的典型正面/负面/中立目标。
One computer science initiative has attempted to identify various emotions in text, focussing on the six so-called basic emotions (Ekman, 1992; Fox, 2008) of anger, disgust, fear, joy, sadness and surprise (Strapparava & Mihalcea, 2008). This initiative also measured emotion strength. A human-annotated corpus was used with the coders allocating a strength from 0 to 100 for each emotion to each text (a news headline), although inter-annotator agreement was low (Pearson correlations of 0.36 to 0.68, depending on the emotion). A variety of algorithms were subsequently trained on this data set. For example, one used WordNet Affect lists to generate appropriate dictionaries for the six emotions. A second approach used a Naive Bayes classifier trained on sets of LiveJournal blogs annotated by their owners with one of the six emotions. The best system (for fine-grained evaluation) was one previously designed for newspaper headlines, UPAR7 (Chaumartin, 2007), which used linguistic parsing and tagging as well as WordNet, SentiWordNet and WordNet Affect, hence relying upon reasonably correct standard grammar and spelling.
一项计算机科学计划试图识别文本中的各种情绪,重点关注六种所谓的基本情绪(Ekman,1992 年;Fox,2008 年),即愤怒、厌恶、恐惧、喜悦、悲伤和惊讶(Strapparava & Mihalcea,2008 年) . 这一举措还衡量了情绪强度。 人工注释的语料库与编码人员一起使用,为每个文本(新闻标题)的每种情绪分配从 0 到 100 的强度,尽管注释者之间的一致性很低(Pearson 相关系数为 0.36 到 0.68,具体取决于情绪)。 随后在该数据集上训练了多种算法。 例如,有人使用 WordNet 情感列表为六种情绪生成适当的词典。 第二种方法使用在 LiveJournal 博客集上训练的朴素贝叶斯分类器,这些博客由其所有者用六种情绪中的一种进行注释。 最好的系统(用于细粒度评估)是以前为报纸头条设计的系统 UPAR7(Chaumartin,2007),它使用语言解析和标记以及 WordNet、SentiWordNet 和 WordNet Affect,因此依赖于合理正确的标准语法和拼写 .
In psychology, the term mood refers to medium and long term affective states. Some blogs and social network sites allow members to describe their mood at the time of editing their status or writing a post, typically by selecting from a range of icons. The results can be used as annotated mood corpora. In theory such corpora ought to be usable to train classifiers to identify mood from the text associated with the mood icon and one system has been designed to do this, but with limited success, probably because the texts analysed are typically short (average 200 words) and there are many moods, some of which are very similar to each other, although even a binary categorisation task also had limited success (Mishne, 2005). A follow up project attempted to derive the proportion of posts with a given mood within a specific time period using 199 words (1-grams) and word pairs (2-grams) derived from the aggregate of all texts, rather than by classifying individual texts (Mishne & de Rijke, 2006). The results showed a high correlation with aggregate self-reported mood. A similar aggregation approach has been applied subsequently in a range of social science contexts (Hopkins & King, 2010).
在心理学中,情绪一词指的是中期和长期的情感状态。 一些博客和社交网站允许会员描述他们在编辑状态或撰写帖子时的心情,通常是从一系列图标中进行选择。 结果可以用作带注释的情绪语料库。 从理论上讲,这样的语料库应该可以用来训练分类器从与情绪图标相关的文本中识别情绪,并且已经设计了一个系统来做到这一点,但收效有限,可能是因为分析的文本通常很短(平均 200 个单词) 并且有很多情绪,其中一些情绪彼此非常相似,尽管即使是二元分类任务也取得了有限的成功(Mishne,2005)。 一个后续项目试图使用从所有文本的集合中派生的 199 个单词(1-grams)和单词对(2-grams)来推导出特定时间段内具有给定情绪的帖子的比例,而不是通过对单个文本进行分类 (Mishne & de Rijke, 2006)。 结果显示与总体自我报告的情绪高度相关。 随后在一系列社会科学背景下应用了类似的聚合方法(Hopkins & King,2010)。
Linguistic processing has also been combined with a pre-existing large collection of subjective common sense statement patterns and applied to relatively informal and domain-independent text in email messages to detect multiple emotions (Liu, Lieberman, & Selker, 2003). This was part of an email support system, however, and the accuracy of the emotion detection was not directly evaluated.
语言处理还与预先存在的大量主观常识陈述模式相结合,并应用于电子邮件消息中相对非正式和领域独立的文本,以检测多种情绪(Liu、Lieberman 和 Selker,2003 年)。 然而,这是电子邮件支持系统的一部分,并且没有直接评估情绪检测的准确性。
Sentiment strength detection
In addition to the research discussed above concerning strength detection for multiple emotions (Strapparava & Mihalcea, 2008), there is some work on positive-negative sentiment strength detection. One previous study used modified sentiment analysis techniques to predict the strength of human ratings on a scale of 1 to 5 for movie reviews (Pang & Lee, 2005). This is a kind of sentiment strength evaluation with a combined scale for positive and negative sentiment. Experiments with human judgements led the authors to merge two of the categories and so the final task was a 4 category classification, with a 3 category version also constructed for testing purposes. A comparison of multi-class SVM classification with SVM regression suggested that SVM regression worked slightly better than multi-class SVM classification when all 4 categories were used but not when only 3 categories were used. It seems likely that the relative performance of SVM regression would increase further as the number of categories increases because the ordering of the classes is implicit information that the multi-class SVM does not use but that SVM regression does. Slight improvements were also gained when information about the percentage of positive sentences in each review was added. This may not be relevant to corpora of very short texts, however.
除了上面讨论的关于多种情绪强度检测的研究 (Strapparava & Mihalcea, 2008),还有一些关于正负情绪强度检测的工作。 之前的一项研究使用改进的情感分析技术来预测人类对电影评论的评分强度,评分范围为 1 到 5(Pang & Lee,2005 年)。 这是一种情绪强度评估,具有正面和负面情绪的组合尺度。 人类判断实验导致作者合并了两个类别,因此最终任务是 4 类别分类,还构建了 3 类别版本用于测试目的。 多类 SVM 分类与 SVM 回归的比较表明,当使用所有 4 个类别时,SVM 回归的效果略好于多类 SVM 分类,但仅使用 3 个类别时则不然。 随着类别数量的增加,SVM 回归的相对性能似乎可能会进一步提高,因为类别的排序是多类别 SVM 不使用但 SVM 回归使用的隐含信息。 当添加有关每个评论中正面句子百分比的信息时,也获得了轻微的改进。 然而,这可能与非常短的文本语料库无关。
Sentiment strength classification has also been developed for a three level scheme (low, medium, and high or extreme) for subjective sentences or clauses in newswire texts using a linguistic analysis converting sentences into dependency trees reflecting their structure (Wilson et al., 2006). Adding dependency trees to unigrams substantially improved the performance of various classifiers compared to unigrams alone, perhaps helped by the fairly large training set (9,313 sentences), the (presumably) good quality grammar of the texts, and the fairly low initial performance on this task (34.5% to 50.9% for unigrams, rising to 48.3% to 55.0% for the three types of classifier applied to level 1 clauses). Here, SVM regression was outperformed by both the rule-based learning Ripper (Cohen, 1995) and BoosTexter, a boosting algorithm combining multiple weak classifiers (Schapire & Singer, 2000).
还针对新闻专线文本中的主观句子或从句开发了三级方案(低、中、高或极端)的情感强度分类,使用语言分析将句子转换为反映其结构的依赖树(Wilson 等人,2006 年) . 与单独的 unigrams 相比,将依赖树添加到 unigrams 大大提高了各种分类器的性能,这可能得益于相当大的训练集(9,313 个句子)、(可能)高质量的文本语法以及此任务的相当低的初始性能 (unigrams 为 34.5% 至 50.9%,应用于 1 级子句的三种分类器上升至 48.3% 至 55.0%)。 在这里,SVM 回归的表现优于基于规则的学习 Ripper (Cohen, 1995) 和 BoosTexter,这是一种结合了多个弱分类器的增强算法 (Schapire & Singer, 2000)。
Quite similar to the current paper is one that measured multiple emotions and their strengths in informal text associated with a dialog system using a combination of methods, including seeking symbolic cues via repeated punctuation (e.g., !!), emoticons and capital letters as well as translating abbreviations (Neviarouskaya, Prendinger, & Ishizuka, 2007). The system also measured emotion intensity on a scale of 0-1 and used a dictionary of terms and intensity ratings assigned by three human judges (with moderate agreement rates: Fleiss Kappa 0.58). The reported evaluation on 160 human-coded sentences showed that in 68% of sentences the system agreed with the coder average to within 20%.
与目前的论文非常相似的是,它使用多种方法测量与对话系统相关的非正式文本中的多种情绪及其强度,包括通过重复标点符号(例如,!!)、表情符号和大写字母以及 翻译缩写 (Neviarouskaya, Prendinger, & Ishizuka, 2007)。 该系统还测量了 0-1 范围内的情绪强度,并使用了由三名人类评委指定的术语和强度评级词典(具有中等一致性率:Fleiss Kappa 0.58)。 报告的对 160 个人工编码句子的评估表明,在 68% 的句子中,系统与编码器的平均一致性在 20% 以内。
Data Set and Human Judgement of Sentiment Strength
MySpace was chosen as a source of test data for this study because it is a public environment containing a large quantity of informal text language and is important in its own right as one of the most visited web sites in the world in 2009. A random sample of MySpace comments was taken by examining the profiles of every 15th member that joined on June 18, 2007, up to 40,000 and selecting those with a declared U.S. nationality and a public profile not of a musician, comedian or film-maker. Of these, those with less than two friends or no comments were rejected as inactive and those with over 1,000 friends or 4,000 comments were rejected as abnormal. A commenting friend was then identified for each remaining member, satisfying the same criteria above, and a random comment selected from each direction of communication between the two. The comments were extracted in December 2008. This produced a large essentially random sample of U.S. commenter-commentee messages. Spam comments and chain messages were subsequently eliminated, as were comments containing images.
之所以选择 MySpace 作为本研究的测试数据源,是因为它是一个包含大量非正式文本语言的公共环境,并且作为 2009 年世界上访问量最大的网站之一,它本身就很重要。随机样本 的 MySpace 评论是通过检查 2007 年 6 月 18 日加入的每 15 名成员的个人资料(最多 40,000 人)并选择那些具有已宣布的美国国籍并且不是音乐家、喜剧演员或电影制作人的公众形象的人来获取的。 其中,好友数少于 2 人或无评论者被视为不活跃被拒绝,好友数超过 1000 人或评论数超过 4000 人被视为异常被拒绝。 然后为每个剩余的成员确定一个评论朋友,满足上述相同的标准,并从两者之间的每个通信方向中选择一个随机评论。 这些评论是在 2008 年 12 月提取的。这产生了美国评论者-被评论者消息的大量随机样本。 垃圾评论和连锁消息随后被删除,包含图像的评论也是如此。
Although sentiment analysis is normally concerned with opinions (Pang & Lee, 2008), Wilson (2008) has generalised this to the psychological task of identifying the author's hidden internal state from their text. For the MySpace data, the objective was not to determine opinions or the author's internal state, however, but to identify the role of expressed sentiment for online communication. Hence the focus of the task was to identify the sentiment expressed in each message, whether reflecting the author's hidden internal state, the intended message interpretation, or the reader's hidden internal state.
尽管情感分析通常与观点有关 (Pang & Lee, 2008),Wilson (2008) 将其概括为从文本中识别作者隐藏的内部状态的心理任务。 然而,对于 MySpace 数据,目标不是确定观点或作者的内部状态,而是确定表达的情绪对在线交流的作用。 因此,任务的重点是识别每条消息中表达的情感,无论是反映作者隐藏的内部状态、预期的消息解释,还是读者隐藏的内部状态。
In order to obtain reliable human judgements of a random sample of the MySpace comments, two pilot exercises were undertaken with separate samples of the data (a total of 2,600 comments). These were used to identify key judgement issues and an appropriate scale. Although there are many ways to measure emotion (Mauss & Robinson, 2009; Wiebe, Wilson, & Cardie, 2005), human coder subjective judgements were used as an appropriate way to gather sufficient results. A set of coder instructions was drafted and refined and an online system constructed to randomly select comments and present them to the coders. One of the key outcomes from the pilot exercise was that the coders treated expressions of energy as expressions of positive sentiment unless in an explicitly negative context. For example, “Hey!!!” would be interpreted as positive because it expresses energy in a context that gives no clue as to the polarity of the emotion, so it would be accepted by most coders as positive by default. In contrast, “Loser!!!” would be interpreted as more negative than “Loser” as the exclamation marks are associated with a negative word. Consequently, the instructions were revised to explicitly state that this conflation of ostensibly neutral energy and positive sentiment was permissible.
为了对 MySpace 评论的随机样本获得可靠的人为判断,我们对不同的数据样本(总共 2,600 条评论)进行了两次试点练习。 这些被用来确定关键的判断问题和适当的规模。 尽管有很多方法可以衡量情绪(Mauss & Robinson,2009;Wiebe、Wilson 和 Cardie,2005),人类编码员的主观判断被用作收集足够结果的适当方法。 起草和完善了一套编码员说明,并构建了一个在线系统来随机选择评论并将其呈现给编码员。 试点工作的主要成果之一是,编码人员将能量表达视为积极情绪的表达,除非是在明确消极的背景下。 例如,“嘿!!!” 将被解释为积极的,因为它在不提供情绪极性线索的上下文中表达能量,因此默认情况下它会被大多数编码员接受为积极的。 相反,“失败者!!!” 会被解释为比“失败者”更消极,因为感叹号与否定词相关联。 因此,对说明进行了修改,明确指出这种表面上中性能量和积极情绪的混合是允许的。
For the final judgements, over a thousand MySpace comments in the data set (20 words and 101 characters per comment, on average) were selected to be judged on a 5 point scale as follows for both positive and negative sentiment.
对于最终判断,选择了数据集中超过 1000 条 MySpace 评论(平均每条评论 20 个单词和 101 个字符),按照以下 5 分制对正面和负面情绪进行判断。
[no positive emotion or energy] 1– 2 – 3 – 4 – 5 [very strong positive emotion]
[no negative emotion] 1– 2 – 3 – 4 – 5 [very strong negative emotion]
The coders were given verbal instructions for coding each text as well as a booklet explaining the task (motivated by Wiebe et al., 2005), with the key instructions reproduced in this article’s appendix. The booklet also contained a list of emoticons and acronyms with explanations and background context of the task for motivation purposes. An early version of the booklet included examples of comments with associated positive and negative sentiment judgements but these had little impact in practice on coders during the pilot testing phase. The set of examples was therefore not used so that inter-coder reliability could be more realistically assessed without the possibility that some of the comments were too similar to the examples given.
编码员得到了对每个文本进行编码的口头说明以及解释任务的小册子(由 Wiebe 等人提出,2005 年),本文附录中转载了关键说明。 这本小册子还包含一系列表情符号和首字母缩略词,以及用于激励目的的任务的解释和背景上下文。 这本小册子的早期版本包括带有相关正面和负面情绪判断的评论示例,但这些在试点测试阶段对编码员的实践影响不大。 因此,没有使用这组示例,以便可以更真实地评估编码员之间的可靠性,而不会出现某些评论与给出的示例过于相似的可能性。
Emotions are perceived differently by individuals, partly because of their life experiences and partly because of personality issues (Barrett, 2006) and gender (Stoppard & Gunn Gruchy, 1993). For system development, the judgements should give a consistent perspective on sentiment in the data, rather than an estimate of the population average perception. As a result, a set of same gender (female) coders was used and initial testing conducted to identify a homogeneous subset. Five coders were initially selected but two were subsequently rejected for giving anomalous results: one gave much higher positive scores than the others, and another gave generally inconsistent results. The mean of the three coders’ results was calculated for each comment and rounded. This was the gold standard for the experiments. Below are some examples of texts and judgements.
个人对情绪的感知不同,部分原因在于他们的生活经历,部分原因在于性格问题 (Barrett, 2006) 和性别问题 (Stoppard & Gunn Gruchy, 1993)。 对于系统开发,判断应该对数据中的情绪给出一致的观点,而不是对人口平均感知的估计。 因此,使用了一组相同性别(女性)的编码员并进行了初步测试以识别同质子集。 最初选择了五名编码员,但后来有两名因给出异常结果而被拒绝:一名给出的积极分数比其他人高得多,而另一名则给出了普遍不一致的结果。 计算每条评论的三位编码员结果的平均值并四舍五入。 这是实验的黄金标准。 下面是一些文本和判断的例子。
- hey witch wat cha been up too (scores: +ve: 2,3,1; -ve: 2,2,2)
- omg my son has the same b-day as you lol (scores: +ve: 4,3,1; -ve: 1,1,1)
- HEY U HAVE TWO FRIENDS!! (scores: +ve: 2,3,2; -ve: 1,1,1)
- What's up with that boy Carson? (scores: +ve: 1,1,1; -ve: 3,2,1)
Table 1 reports the degree of inter-coder agreement. Basic agreement rates are reported here for comparability with SentiStrength. Previous emotion-judgement/annotation tasks have obtained higher inter-coder scores, but without strength measures and therefore having fewer categories (e.g., Wiebe et al., 2005). Moreover, one previous paper noted that inter-coder agreement was higher on longer (blog) texts (Gill, Gergle, French, & Oberlander, 2008), suggesting that obtaining agreement on the short texts here would be difficult. The appropriate type of inter-coder reliability statistic for this kind of data with multiple coders and varying differences between categories is Krippendorff’s α (Artstein & Poesio, 2008; Krippendorff, 2004). Using the numerical difference in emotion score as weights, the three coder α values were 0.5743 for positive and 0.5634 for negative sentiment. These values are positive enough to indicate that there is broad agreement between the coders but not positive enough (e.g., < 0.67. although precise limits are not applicable to Krippendorff’s α with weights) to suggest that the coders are consistently measuring a clear underlying construct. Nevertheless, using the average of the coders as the gold standard still seems to be a reasonable method to get sentiment strength estimates.
表 1 报告了编码器间的一致性程度。 此处报告的基本一致率是为了与 SentiStrength 进行比较。 以前的情绪判断/注释任务获得了更高的编码间分数,但没有强度测量,因此类别较少(例如,Wiebe 等人,2005)。 此外,之前的一篇论文指出,编码员之间对较长(博客)文本的一致性更高(Gill、Gergle、French 和 Oberlander,2008 年),这表明在这里就短文本达成一致是很困难的。 对于此类具有多个编码器和不同类别之间差异的数据,合适的编码器间可靠性统计类型是 Krippendorff 的 α(Artstein & Poesio,2008;Krippendorff,2004)。 使用情绪得分的数值差异作为权重,三个编码器的 α 值对于积极情绪为 0.5743,对于消极情绪为 0.5634。 这些值足以表明编码人员之间存在广泛的一致性,但还不够积极(例如,< 0.67。尽管精确限制不适用于带权重的 Krippendorff α)以表明编码人员始终如一地测量清晰的基础结构。 尽管如此,使用编码器的平均值作为黄金标准似乎仍然是获得情绪强度估计的合理方法。
Table 1. Level of agreement between coders for the 1,041 evaluation comments (exact agreement, % of agreements within one class, mean percentage error, and Pearson correlation).
表 1. 编码员之间对 1,041 条评估意见的一致程度(完全一致、一类内的一致百分比、平均百分比错误和 Pearson 相关)。
Comparison | +ve | +ve +/- 1 class | +ve mean % diff. | +ve corr | -ve | -ve +/- 1 class | -ve mean % diff. | -ve corr |
---|---|---|---|---|---|---|---|---|
Coder 1 vs. 2 | 51.0% | 94.3% | .256 | .564 | 67.3% | 94.2% | .208 | .643 |
Coder 1 vs. 3 | 55.7% | 97.8% | .216 | .677 | 76.3% | 95.8% | .149 | .664 |
Coder 2 vs. 3 | 61.4% | 95.2% | .199 | .682 | 68.2% | 93.6% | .206 | .639 |
The SentiStrength Sentiment Strength Detection Algorithm
The SentiStrength emotion detection algorithm was developed on an initial set of 2,600 MySpace classifications used for the pilot testing. The key elements of SentiStrength are listed below.
SentiStrength 情绪检测算法是在用于试点测试的初始 2,600 个 MySpace 分类集上开发的。 下面列出了 SentiStrength 的关键要素。
- The core of the algorithm is the sentiment word strength list. This is a collection of 298 positive terms and 465 negative terms classified for either positive or negative sentiment strength with a value from 2 to 5. The default classifications are based upon human judgements during the development stage, with automatic modification occurring later during the training phase (see below). Following LIWC, some of the words include wild cards (e.g., xx*) matches any number ≥2 of consecutive xs. Some terms are standard English words and others are non-standard but common in MySpace (e.g., luv, xox, lol, haha, muah). The emotion strength is specific to the contexts in which the words tend to be used in MySpace. For example, “love” was originally classified as strength 4 positive but was reduced to strength 3 due to many casual uses such as “Just showin love 2 ur page”. Some of the words explicitly express emotion, such as “love” or “hate” but others, normally given a weak strength 2, are indirectly associated with positive or negative contexts (e.g., appreciate, help, birthday). The SentiStrength algorithm includes procedures (described below) to fine-tune the sentiment strengths using a set of training data. > 该算法的核心是情感词强度列表。这是一个包含 298 个正面术语和 465 个负面术语的集合,按正面或负面情绪强度分类,值从 2 到 5。默认分类基于 开发阶段的人为判断,稍后在训练阶段进行自动修改(见下文)。 在 LIWC 之后,一些包含通配符的单词(例如 xx*)匹配任意数量 ≥2 的连续 x。 有些术语是标准的英语单词,而另一些是非标准的但在 MySpace 中很常见(例如,luv、xox、lol、haha、muah)。 情感强度特定于 MySpace 中倾向于使用单词的上下文。 例如,“love”最初被归类为强度 4 正面,但由于许多随意使用(例如“Just showin love 2 ur page”)而被降为强度 3。 一些词明确表达情感,例如“爱”或“恨”,但其他词通常具有较弱的强度 2,与积极或消极的语境间接相关(例如,欣赏、帮助、生日)。 SentiStrength 算法包括使用一组训练数据微调情绪强度的过程(如下所述)。
- The above default manual word strengths are modified by a training algorithm to optimise the sentiment word strengths. This algorithms starts with the baseline human-allocated term strengths for the predefined list and then for each term assesses whether an increase or decrease of the strength by 1 would increase the accuracy of the classifications. Any change that increases the overall accuracy by at least 2 is kept. The minimum increase could also be set to 1 which would risk over-fitting, whereas 2 risks loosing useful changes to rare words. Here 2 was selected to make the algorithm run faster, due to less changes, rather than for any theoretical reason (in fact the algorithm worked better on the test data with 1, as the results show). The algorithm tests all words in the sentiment list at random and is repeated until all words have been checked without their strengths being changed. > 上述默认手动词强度由训练算法修改以优化情感词强度。 该算法从预定义列表的基线人工分配术语强度开始,然后针对每个术语评估强度增加或减少 1 是否会提高分类的准确性。 保留至少将整体精度提高 2 的任何更改。 最小增量也可以设置为 1,这会存在过度拟合的风险,而 2 则可能会丢失对稀有词的有用更改。 这里选择 2 是为了让算法运行得更快,因为变化更少,而不是出于任何理论上的原因(事实上,算法在 1 的测试数据上效果更好,如结果所示)。 该算法随机测试情感列表中的所有单词并重复进行,直到检查完所有单词且其强度没有改变。
- The word “miss” was allocated a positive and negative strength of 2. This was the only word classed as both positive and negative. It was typically used in the phrase “I miss you”, suggesting both sadness and love. > “miss”这个词被分配了 2 的正面和负面强度。这是唯一一个同时被归类为正面和负面的词。 它通常用于短语“我想你”中,暗示着悲伤和爱。
- A spelling correction algorithm identifies the standard spellings of words that have been miss-spelled by the inclusion of repeated letters. For example hellllloooo would be identified as “hello” by this algorithm. The algorithm (a) automatically deletes repeated letters above twice (e.g., helllo -> hello); (b) deletes repeated letters occurring twice for letters rarely occurring twice in English (e.g., niice -> nice) and (c) deletes letters occurring twice if not a standard word but would form a standard word if deleted (e.g., nnice -> nice but not hoop -> hop nor baaz -> baz). Formal spelling correction algorithms (see Pollock & Zamora, 1984) were tried but not used as they made very few corrections and had problems with names and slang. > 拼写校正算法识别因包含重复字母而拼写错误的单词的标准拼写。 例如,helllllooooo 将被该算法识别为“hello”。 算法(a)自动删除上面两次重复的字母(例如,hello -> hello); (b) 删除在英语中很少出现两次的字母重复出现两次的字母(例如,niice -> nice)和 (c) 删除出现两次的字母如果不是标准词但如果删除将形成标准词(例如 nnice -> nice) 不错,但不是 hoop -> hop 或 baaz -> baz)。 正式的拼写校正算法(参见 Pollock & Zamora,1984)被尝试但没有被使用,因为它们只做了很少的校正并且在名称和俚语方面存在问题。
- A booster word list contains words that boost or reduce the emotion of subsequent words, whether positive or negative. Each word increases emotion strength by 1 or 2 (e.g., very, extremely) or decreases it by 1 (e.g., some). > 助推词列表包含可以提升或降低后续词的情绪的词,无论是积极的还是消极的。 每个词都会将情绪强度增加 1 或 2(例如,非常、极端)或减少 1(例如,一些)。
- A negating word list contains words that invert subsequent emotion words (including any preceding booster words). For example, if “very happy” had positive strength 4 then “not very happy” would have negative strength 4. The possibility that some negating terms do not negate was not incorporated as this did not seem to occur often in the pilot data set. > 否定词列表包含反转后续情感词(包括任何前面的助推词)的词。 例如,如果“非常高兴”的正强度为 4,那么“不太高兴”的负强度为 4。一些否定词不否定的可能性没有被纳入,因为这在试验数据集中似乎并不经常发生。
- Repeated letters above those needed for correct spelling are used to give a strength boost of 1 to emotion words, as long as there are at least two additional letters. The use of repeated letters is a common device for expressing emotion or energy in MySpace comments, but one repeated letter often appeared to be a typing error. > 重复的字母高于正确拼写所需的字母,用于将情感词的强度提高 1,只要至少有两个额外的字母。 使用重复的字母是 MySpace 评论中表达情感或能量的常用手段,但重复的字母往往看起来像是打字错误。
- An emoticon list with associated strengths (positive or negative 2) supplements the sentiment word strength list (and punctuation included in emoticons is not processed further for the purposes below). > 具有相关强度(正面或负面 2)的表情符号列表补充了情感词强度列表(出于以下目的,不会进一步处理表情符号中包含的标点符号)。
- Any sentence with an exclamation mark was allocated a minimum positive strength of 2. > 任何带有 感叹号 的句子都被分配了最小正强度 2。
- Repeated punctuation including at least one exclamation mark gives a strength boost of 1 to the immediately preceding emotion word (or sentence). > 重复的标点符号包括至少一个感叹号,使紧接在前的情感词(或句子)的强度提高 1。
- Negative emotion was ignored in questions. For example, the question “are you angry?” would be classified as not containing sentiment, despite the presence of the word “angry”. This was not applied to positive sentiment because many question sentences appeared to contain mild positive sentiment. In particular, sentences like “whats up?” were typically classified as containing mild positive sentiment (strength 2). > 问题中忽略了负面情绪。 例如,“你生气了吗?”这个问题。 将被归类为不包含情绪,尽管存在“愤怒”一词。 这不适用于积极情绪,因为许多问题句子似乎包含温和的积极情绪。 特别是像“怎么了?”这样的句子。 通常被归类为包含温和的积极情绪(强度 2)。
The above factors were applied separately to each sentence, with the sentence being assigned with both the most positive and most negative emotion identified in it. Each overall comment was assigned with the most positive of its sentence emotions and the most negative of its sentence emotions. Sentence were split either by line breaks in comments or after punctuation other than emoticons.
上述因素分别应用于每个句子,句子被分配了其中确定的最积极和最消极的情绪。 每个整体评论都分配有最积极的句子情绪和最消极的句子情绪。 句子被评论中的换行符或除表情符号以外的标点符号分隔。
Some additional modifications were added to SentiStrength but subsequently rejected after additional testing, or were found to be impractical.
一些额外的修改被添加到 SentiStrength,但随后在额外测试后被拒绝,或者被发现是不切实际的。
- Phrase identification was not extensively used except for a few frequent examples found in the initial 2,600 development comments. Although idiomatic phrases were common, their variety was such that it did not seem practical to systematically identify them. Future work could perhaps identify booster phrases like “so much” and “a lot”, and use phrase identification to separate weak uses of the word “love” with stronger uses, such as “I love you”. > 除了在最初的 2,600 条开发评论中发现的几个常见示例外,短语识别没有被广泛使用。 尽管惯用语很常见,但它们的多样性使得系统地识别它们似乎并不实用。 未来的工作可能会识别像“这么多”和“很多”这样的助推短语,并使用短语识别来区分“爱”这个词的弱用法和强用法,比如“我爱你”。
- Semantic disambiguation was not used for ambiguous words because of the problems caused by highly non-standard grammar. This could potentially improve the algorithm but would require considerable computational effort. For example, the word “rock” was sometimes strongly positive (e.g., you rock!!!) and sometime neutral (e.g., do you listen to rock music?). > 由于高度不标准的语法引起的问题,语义消歧不用于歧义词。 这可能会改进算法,但需要大量的计算工作。 例如,“摇滚”这个词有时是非常积极的(例如,你摇滚!!!),有时是中性的(例如,你听摇滚音乐吗?)。
Experiments
SentiStrength was tested on a set of 1,041 MySpace comments that were different from the comments used in the development phase and were classified by three people (see Table 1), and the average was used as the gold standard. A 10-fold cross-validation approach was used. The results were compared to random allocation and to the baseline majority class classification (a positive sentiment of 2 and a negative sentiment of 1). SentiStrength was also compared to a range of standard machine learning classification algorithms in Weka (Witten & Frank, 2005) using the frequencies of each word in the sentiment word list as the feature set. The extended feature set used for the comparisons included n-grams of length 1-3 consisting of all terms extracted from the text, including emoticons, spelling-corrected words (where appropriate), repeated punctuation, question marks and exclamation marks (e.g., one feature was the 3-gram: “love-u-!”) as well as counts of the total number of 1, 2, and 3-grams in each comment. This extended set of features incorporates most of the elements of text used by SentiStrength.
A second test compared different feature sets to see whether alternative smaller feature sets could give better results for machine learning and to discover which features were most useful.
A third test used feature reduction with subsumption (see below for details).
A fourth test compared different variations of SentiStrength to see which aspects of the algorithm were most powerful.
Comparison with machine learning, extended feature set
Figures 1 and 2 show the performance of various machine learning algorithms on the 1,041 MySpace comments with different feature set sizes, as selected using the top-ranking features from the information gain metric. Feature selection improved the results for all methods, with one minor exception (Naïve Bayes for positive sentiment: 52.0% without feature selection, averaged over 4 10-fold cross-validations). For each method, Table 2 reports comparisons with SentiStrength using the optimal feature set size for each method.
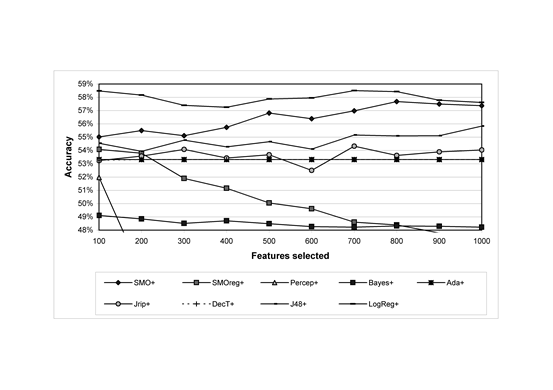
Fig. 1. Positive sentiment classification accuracy against feature set size for different classifiers using the extended feature set; average over 4 classifications.
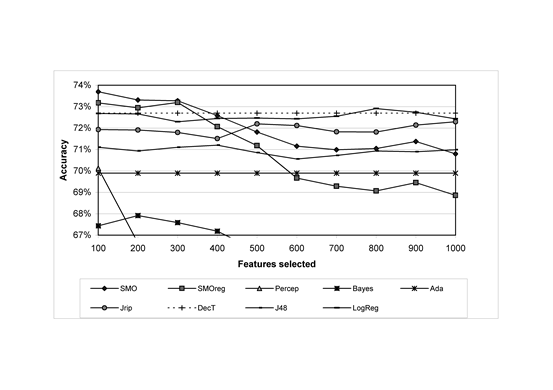
Fig. 2. Negative sentiment classification accuracy against feature set size for different classifiers using the extended feature set; average over 4 classifications.
From Table 2, machine learning classifiers using the extended feature set with the optimal number of features, as selected by information gain, are significantly less accurate than SentiStrength. SentiStrength also has the highest correlation with the gold standard, the lowest mean percentage error and the highest accuracy to within one class. Hence it performs consistently better (at least 2.1%) than the other algorithms. The level of accuracy for SentiStrength is nevertheless moderate at 60.6%. This is similar to the degree of agreement between the human coders (Table 1), suggesting that positive sentiment strength detection in informal short texts is an inherently difficult task.
Table 2. Performance of various algorithms on positive sentiment strength detection for 1,041 comments with the extended feature set and 10-fold cross-validation (decreasing order of positive sentiment strength performance). Other than SentiStrength, results are averages over 4 runs of different random test/training splits and for the optimal feature numbers, as selected from Figure 1.
Algorithm | Optimal features | Accuracy | Accuracy +/- 1 class | Corr. | Mean % absolute error |
---|---|---|---|---|---|
SentiStrength (standard configuration, 30 runs) | - | 60.6% | 96.9% | .599 | 22.0% |
Simple logistic regression | 700 | 58.5% | 96.1% | .557 | 23.2% |
SVM (SMO) | 800 | 57.6% | 95.4% | .538 | 24.4% |
J48 classification tree | 700 | 55.2% | 95.9% | .548 | 24.7% |
JRip rule-based classifier | 700 | 54.3% | 96.4% | .476 | 28.2% |
SVM regression (SMO) | 100 | 54.1% | 97.3% | .469 | 28.2% |
AdaBoost | 100 | 53.3% | 97.5% | .464 | 28.5% |
Decision table | 200 | 53.3% | 96.7% | .431 | 28.2% |
Multilayer Perceptron | 100 | 50.0% | 94.1% | .422 | 30.2% |
Naïve Bayes | 100 | 49.1% | 91.4% | .567 | 27.5% |
Baseline | - | 47.3% | 94.0% | - | 31.2% |
Random | - | 19.8% | 56.9% | .016 | 82.5% |
Bold=sig at 0.01, italic=sig at 0.05 compared to SentiStrength.
For negative sentiment strength, most of the methods give quite similar results and some give better results than SentiStrength. Although the SentiStrength accuracy is 72.8%, this is only 2.9% better than the baseline, several of the other methods have similar levels of accuracy and SVM is significantly more accurate. SentiStrength is significantly the most accurate of the methods if up to one class error is allowed, and has significantly the highest correlation with the human coder results. Note that in theory none of the methods ought to be worse than the baseline but this can occur due to optimisation on the training set rather than the evaluation set. Overall, it seems that SentiStrength is not good at identifying negative emotion but that this is a hard task for the short texts analysed here. Note also that the mean percentage absolute error for the random category is over 100% due to the predominance of ‘1’ as the correct category for negative sentiment.
Table 3. Performance of various algorithms on negative sentiment strength detection for 1,041 comments with the extended feature set and 10-fold cross-validation (decreasing order of positive sentiment strength performance). Other than SentiStrength, results are averages over 4 runs and for the optimal feature numbers, as selected from Figure 2.
Algorithm | Optimal features | Accuracy | Accuracy +/- 1 class | Corr. | Mean % absolute error |
---|---|---|---|---|---|
SVM (SMO) | 100 | 73.5% | 92.7% | .421 | 16.5% |
SVM regression (SMO) | 300 | 73.2% | 91.9% | .363 | 17.6% |
Simple logistic regression | 800 | 72.9% | 92.2% | .364 | 17.3% |
SentiStrength (standard configuration, 30 runs) | - | 72.8% | 95.1% | .564 | 18.3% |
Decision table | 100 | 72.7% | 92.1% | .346 | 17.0% |
JRip rule-based classifier | 500 | 72.2% | 91.5% | .309 | 17.3% |
J48 classification tree | 400 | 71.1% | 91.6% | .235 | 18.8% |
Multilayer Perceptron | 100 | 70.1% | 92.5% | .346 | 20.0% |
AdaBoost | 100 | 69.9% | 90.6% | - | 16.8% |
Baseline | - | 69.9% | 90.6% | - | 16.8% |
Naïve Bayes | 200 | 68.0% | 89.8% | .311 | 27.3% |
Random | - | 20.5% | 46.0% | .010 | 157.7% |
Bold=sig at 0.01, italic=sig at 0.05 compared to SentiStrength.
The remainder of the paper focuses on positive sentiment alone, since the results for negative sentiment are not significant.
Comparison of feature sets for machine learning –positive sentiment strength
Figures 3 and 4 compare the impact of using different feature sets with the two best-performing algorithms for positive sentiment strength detection. The feature sets are: 1-3-grams; 1-3-grams with emoticons; 1-3-grams with punctuation; 1-3-grams with misspellings (i.e., including terms before spelling correction in addition to terms after spelling correction, when different); 1-3-grams with emoticons, punctuation and misspellings; 1-3-grams with emotion terms; and 1-grams. The basic bag or words approach (1-grams) performs poorly – always the worst feature set for logistic regression and the worst or amongst the worst few feature sets all the time for SVM. For SVM, the best results are achieved with the basic 1-3-grams enhanced by the emotion terms, although most of the time (i.e., for 500-1000 features) the extended feature set (labelled “all of the above” and the same as used in the results above) performs best, perhaps mainly due to the punctuation component, since this enhancement performs second best for 700-1000 features.
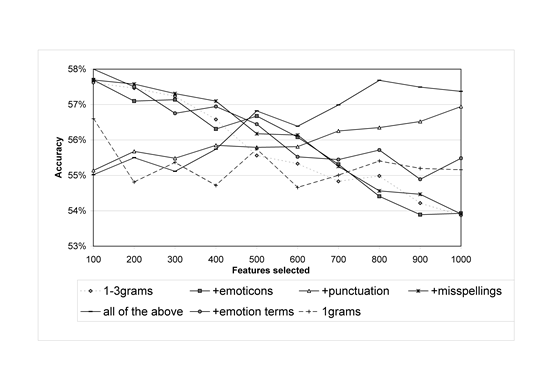
Fig. 3. SVM (SMO) positive sentiment classification accuracy against feature set size for different feature set types; average over 4 classifications.
Figure 4 suggests that, other than the basic bag of words, the difference between feature sets is less clear-cut for logistic regression than for SVM but the best performing combination is again the 1-3 grams plus emotion terms. For larger feature sets, the combined feature set performed best, probably due to the punctuation and emotion terms.

Fig. 4. Logistic regression positive sentiment classification accuracy against feature set size for different feature set types; average over 4 classifications.
A potential weakness of using bigrams and trigrams in conjunction with unigrams is that there is some redundancy involved. For instance, the trigram “I love you” will also match the bigrams “I love” and “love you” as well as the unigrams “I”, “love” and “you”. In response, subsumption is a feature selection method that eliminates bigrams and trigrams that appear to be redundant in the sense of not giving additional information above that of their constituent unigrams (and bigrams for trigrams). This approach is appropriate here. Subsumption was applied with a logical extension: that word patterns, like happ* could eliminate matching words (e.g., happily, happy in this case) if the appropriate measure was matched. Figures 5 and 6 show the results of subsumption for the two machine learning algorithms for which it performed best: SVM and logistic regression. Subsumption performs best in conjunction with feature reduction, as both graphs show. For the other algorithms, subsumption improved the performance of Jrip by 0.4% (α = 0.005, 100 features), SVM regression by 1.1% (α = 0.02, 100 features), multilayer perceptron by 1.0% (α = 0.02, 100 features) and decision table by 1.0% (α = 0.005, 900 features) but did not improve J48, AdaBoost and Naïve Bayes.
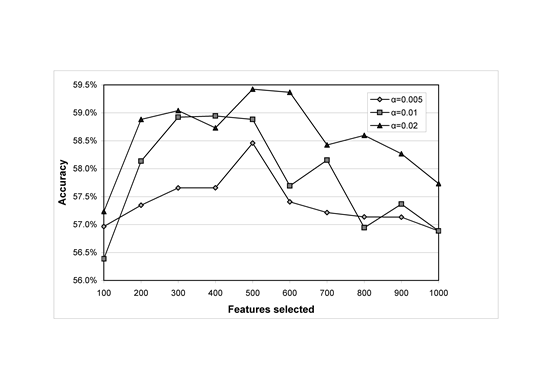
Fig. 5. SVM(SMO) positive sentiment classification accuracy against feature set size for subsumption with various α values; average over 5 classifications.
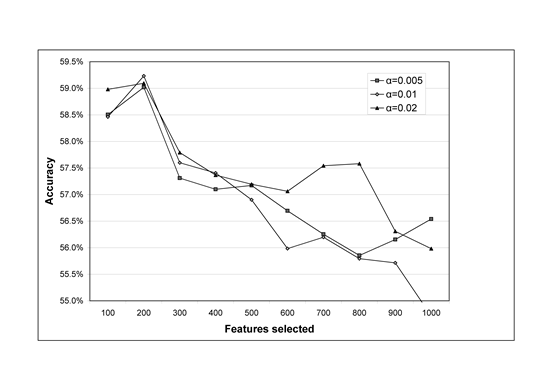
Fig. 6. Logistic regression positive sentiment classification accuracy against feature set size for subsumption with various α values; average over 5 classifications.
From Figure 5, SVM with subsumption outperforms SVM without subsumption on the extended feature set by 1.8%, and outperforms SVM on all the other feature sets (α = 0.02, 500 features). Nevertheless, its accuracy is lower than the SentiStrength standard version, although the difference is not statistically significant (accuracy = 59.42%, accuracy +/-1 = 96.60%, correlation = 0.5822, mean absolute error = 22.65%; only the mean absolute error difference is statistically significant from SentiStrength standard configuration). From Figure 6, logistic regression with subsumption outperforms logistic regression without on the extended feature set by a lower margin of 0.7% (α = 0.01, 200 features). It performs less well than 1-3grams with the emotion terms added, however (Figure 4), but this could be a statistical anomaly due to the large number of comparisons performed. Logistic regression performs less well than standard SentiStrength, but the difference is again not significant (accuracy = 59.23%, accuracy +/-1 = 95.79%, correlation = 0.5820, mean absolute error = 22.57%; all except accuracy are statistically significantly different from SentiStrength standard configuration). In terms of α values, 0.02 tends to perform almost uniformly better than other values for this data set.
Note that that although SentiStrength is not statistically significantly better than the optimal SVM and logistic regression models using subsumption, the optimal variation of SentiStrength in Table 4, with one simple modification (training needs only increase of 1 to alter word strengths), is statistically significantly better in all respects than SVM and is statistically significantly better in all respects, except accuracy within +/-1, than logistic regression.
Comparison of SentiStrength versions
Tables 4 and 5 report comparisons of different variations of SentiStrength. Most variations have little influence on the results – individually accounting for a maximum of 0.8% of the performance of the algorithm, except for the last two options. These differences are small enough to be attributable to the corpus used and so the table does not provide convincing evidence that any of the variations are better or worse than the standard approach. When removing all the options (but not changing the averaging method) the cumulative effect is more significant, however, reducing performance by 3.4%. Perhaps comments using non-standard features tend to use multiple non-standard features and so if one special rule is ignored then this is frequently compensated for by the other special rules.
Compared with tables 2 and 3, the main power of SentiStrength is in the combined effect of its rules to adapt to various informal text variations as well as in the overall approach of using a list of term strengths and identifying the strongest positive and negative terms in any comment. In this context, it seems that the generic classification algorithms in Table 2 were a minimum of 2.1% less effective than SentiStrength mainly due to the 1-3 grams approach being insufficiently flexible to cope with non-standard MySpace language (about 3.4% attributable to this cause). In addition, it seems that they were not able to draw upon a large enough training set to learn effective term strengths and a much larger training set could see some of them approach closer to the performance of SentiStrength. Finally, note that the variations of SentiStrength that apparently improve it are not robustly better: when all these are combined to make a new version of SentiStrength this has exactly the same accuracy as the standard configuration (60.64% correct, 97.07% +/- 1 class, .6071 correlation, 21.62% mean % error).
Table 4. Comparison of the positive emotion performance over several algorithm variations: average over 30 10-fold cross-validations for 1,041 classified comments.
Type | % Correct | +/- 1 class | corr. | Mean % err. (pred-act)/act |
---|---|---|---|---|
SentiStrength standard algorithm (but training needs only increase of 1 to alter word strengths) | 61.03% | 96.68% | .5983 | 21.66% |
Negating words not used to switch following sentiment (e.g., not happy) | 60.87% | 97.50% | .6206 | 21.28% |
Multiple consecutive positive words not used as emotion boosters | 60.70% | 96.88% | .5962 | 21.97% |
Emoticons ignored | 60.68% | 96.87% | .5977 | 21.95% |
Booster words ignored (e.g., very) | 60.68% | 96.80% | .5970 | 22.14% |
SentiStrength standard algorithm | 60.64% | 96.90% | .5986 | 21.96% |
Exclamation marks not given a strength of 2 | 60.51% | 96.62% | .6035 | 21.47% |
Automatic spelling correction disabled | 60.39% | 96.88% | .5961 | 22.05% |
Extra multiple letters not used as emotion boosters | 60.21% | 96.81% | .5952 | 22.16% |
The term “miss” not given a strength of +2 | 60.45% | 96.77% | .5953 | 22.16% |
Idiom lookup table disabled | 60.52% | 96.88% | .6054 | 21.62% |
Neutral words with emphasis not counted as positive emotion | 60.13% | 96.79% | .5966 | 21.90% |
SentiStrength with all the above changes | 57.44% | 96.07% | .6073 | 21.91% |
Sentence sentiment is the average of all term sentiments (rather than the maximum) | 42.40% | 88.54% | .4065 | 29.27% |
Text sentiment is the average of all sentence sentiments (rather than the maximum) | 39.13% | 86.96% | .3293 | 33.19% |
* Bold=significant at p=0.01, italic=sig. at p=0.05, compared to the standard algorithm.
Table 5. Comparison of the negative emotion performance over several algorithm variations: average over 30 10-fold cross-validations for 1,041 classified comments.
Type | % Correct | +/- 1 class | corr. | Mean % err. (pred-act)/act |
---|---|---|---|---|
Negative sentiment in questions is not ignored | 73.56% | 95.14% | .5921 | 18.11% |
SentiStrength standard algorithm (but training needs only increase of 1 to alter word strengths) | 72.95% | 94.86% | .5651 | 18.16% |
Negating words not used to switch following sentiment (e.g., not happy) | 72.84% | 94.79% | .5706 | 18.35% |
SentiStrength standard algorithm | 72.83% | 95.07% | .5644 | 18.27% |
Multiple consecutive negative words not used as emotion boosters | 72.81% | 95.08% | .5653 | 18.29% |
Emoticons ignored | 72.80% | 94.97% | ._5614_ | 18.28% |
SentiStrength with all the changes in this table except averaging | 72.76% | 94.59% | .5668 | 19.07% |
Idiom lookup table disabled | 72.73% | 95.03% | .5556 | 18.63% |
Extra multiple letters not used as emotion boosters | 72.72% | 95.04% | .5627 | 18.40% |
Text sentiment is the average of all sentence sentiments (rather than the maximum) | 72.66% | 95.83% | .5486 | 16.81% |
Automatic spelling correction disabled | 72.64% | 95.07% | .5586 | 18.62% |
Booster words ignored (e.g., very) | 72.35% | 95.03% | .5559 | 18.50% |
Sentence sentiment is the average of all term sentiments (rather than the maximum) | 72.17% | 95.35% | .4980 | 16.82% |
* Bold=significant at p=0.01, italic=sig.t at p=0.05, compared to the standard algorithm.
Table 5 shows that there is very little variation in the performance of the different variations of SentiStrength for negative emotion strength detection: the performance differs from the standard configuration by a maximum of 0.83%. It suggests however, that negative sentiment in questions (e.g., “Do you hate Tony?”) should not be ignored in future.
Discussion and Conclusions
Recall that the main novel contributions of this paper are: a machine learning approach to optimise sentiment term weightings; methods for extracting sentiment from non-standard spelling in text; and a related spelling correction method. SentiStrength was able to identify the strength of positive sentiment on a scale of 1 to 5 in 60.6% of the time in informal MySpace language, significantly above the best standard machine-learning approaches which had a performance of up to 58.5% - in line with those for a previous 4-category opinion intensity classification task (Wilson et al., 2006). The standard version of SentiStrength was also better then standard machine learning methods when their performance was improved (or not, in some cases) with the use of subsumption and information gain feature reduction, but the difference was not statistically significant. A slightly modified version of SentiStrength was statistically significantly better than the improved machine learning methods, however. This is good evidence of the efficacy of SentiStrength for positive sentiment strength detection given the range of different algorithms and parameters that it was compared against (9 algorithms x 11 feature set sizes, x 7 feature set types = 693 variations, plus 9 algorithms x 10 feature set sizes x 3 α values = 270 variations for subsumption), which gives lower-performing algorithms a reasonable statistical chance of outperforming SentiStrength through chance, but none did.
The main reason for SentiStrength’s relative success seems to be procedures for decoding non-standard spellings and methods for boosting the strength of words, which accounted for much of its performance. Without these factors, the SentiStrength variant based solely upon a dictionary of emotion-associated words and their estimated strengths with 57.5% was only 1.3% better than the most successful machine learning approach on an extended set of 1-3grams. In contrast, SentiStrength was able to identify negative sentiment little better (1.8%) than the baseline, probably due to creativity in expressing negative comments or due to the difficulty in getting significantly above the baseline when one category dominates (Artstein & Poesio, 2008; Krippendorff, 2004). It seems that both positive and negative sentiment detection in informal text language like MySpace comments is challenging because of several factors: language creativity, expressions of sentiment without emotion-bearing words, and differences between human coder interpretations meaning that there is not a genuinely correct classification for most comments.
Given the success in generating an algorithm for positive sentiment strength detection and the predominance of positive sentiment in MySpace comments, it seems that future research can apply the sentiment strength detection techniques to automatically identify and classify positive sentiment in informal web communication environments on a large scale. Moreover, there are many commercial applications of sentiment analysis, some of which use informal computer text generate from chatrooms or mobile phone text messages, and this algorithm shows that it is possible to estimate the strength of positive sentiment even in these short messages.
In terms of future work, a next logical step is to attempt to improve the performance of the system through linguistic processing, despite the poor grammar of the short informal text messages analysed. Previous work has shown that this approach is promising, particularly via dependency trees (Wilson et al., 2009) and that, given a large enough training sample, improvements may be possible even in poor quality text (Gamon, 2004).
Appendix: Coder Instructions (extract)
Code each comment for the degree to which it expresses positive emotion or energy. Excitement, enthusiasm or energy should be counted as positive emotion here. If you think that the punctuation emphasises the positive emotion or energy in any way then include this in your rating. The scale for *positive* emotion or energy is:
[no positive emotion or energy] 1– 2 – 3 – 4 – 5 [very strong positive emotion]
- Allocate 1 if the comment contains no positive emotion or energy.
- Allocate 5 if the comment contains very strong positive emotion.
- Allocate a number between 2 and 4 if the comment contains some positive emotion but not very strong positive emotion. Use your judgement about the exact positive emotion strength.
Code each comment for the degree to which it expresses negative emotion or is negative. If you think that the punctuation emphasises the negative emotion in any way then include this in your rating. The scale for *negative* emotion is:
[no negative emotion] 1– 2 – 3 – 4 – 5 [very strong negative emotion]
- Allocate 1 if the comment contains no negative emotion at all.
- Allocate 5 if the comment contains very strong negative emotion.
- Allocate a number between 2 and 4 if the comment contains some negative emotion but not very strong negative emotion. Use your judgement about the exact negative emotion strength.
When making judgements, please be as consistent with your previous decisions as possible. Also, please interpret emotion within the individual comment that it appears and ignore all other comments.
References
Abbasi, A., Chen, H., & Salem, A. (2008). Sentiment analysis in multiple languages: Feature selection for opinion classification in web forums. ACM Transactions on Information Systems, 26(3), 12:11-12.34.
Abbasi, A., Chen, H., Thoms, S., & Fu, T. (2008). Affect analysis of Web forums and Blogs using correlation ensembles. IEEE Transactions on Knowledge and Data Engineering, 20(9), 1168-1180.
Agerri, R., & García-Serrano, A. (2010). Q-WordNet: Extracting polarity from WordNet senses. Proceedings of the Seventh conference on International Language Resources and Evaluation, Retrieved May 25, 2010 from: http://www.lrec-conf.org/proceedings/lrec2010/pdf/2695_Paper.pdf.
Argamon, S., Whitelaw, C., Chase, P., Hota, S. R., Garg, N., & Levitan, S. (2007). Stylistic text classification using functional lexical features. Journal of the American Society for Information Science and Technology, 58(6), 802-822.
Artstein, R., & Poesio, M. (2008). Inter-coder agreement for computational linguistics. Journal of Computational Linguistics, 34(4), 555-596.
Baccianella, S., Esuli, A., & Sebastiani, F. (2010). SentiWordNet 3.0: An enhanced lexical resource for sentiment analysis and opinion mining. Proceedings of the Seventh conference on International Language Resources and Evaluation, Retrieved May 25, 2010 from: http://www.lrec-conf.org/proceedings/lrec2010/pdf/2769_Paper.pdf.
Balahur, A., Kozareva, Z., & Montoyo, A. (2009). Determining the polarity and source of opinions expressed in political debates. Lecture Notes in Computer Science, 5449, 468-480.
Balahur, A., Steinberger, R., Kabadjov, M., Zavarella, V., Goot, E. v. d., Halkia, M., et al. (2010). Sentiment analysis in the news. Proceedings of the Seventh conference on International Language Resources and Evaluation, Retrieved May 25, 2010 from: http://www.lrec-conf.org/proceedings/lrec2010/pdf/2909_Paper.pdf.
Baron, N. S. (2003). Language of the Internet. In A. Farghali (Ed.), The Stanford Handbook for Language Engineers (pp. 59-127). Stanford: CSLI Publications.
Barrett, L. F. (2006). Valence as a basic building block of emotional life. Journal of Research in Personality, 40(1), 35-55.
boyd, d. (2008). Taken out of context: American teen sociality in networked publics. University of California, Berkeley, Berkeley.
boyd, d. (2008). Why youth (heart) social network sites: The role of networked publics in teenage social life. In D. Buckingham (Ed.), Youth, identity, and digital media (pp. 119-142). Cambridge, MA: MIT Press.
Brill, E. (1992). A simple rule-based part of speech tagger. Proceedings of the Third Conference on Applied Natural Language Processing, 152-155.
Chaumartin, F.-R. (2007). UPAR7: A knowledge-based system for headline sentiment tagging. In Proceedings of the 4th International Workshop on Semantic Evaluations (SemEval-2007) (pp. 422-425). New York, NY: ACM.
Choi, Y., & Cardie, C. (2008). Learning with compositional semantics as structural inference for subsentential sentiment analysis. Proceedings of the Conference on Empirical Methods in Natural Language Processing, 793-801.
Cohen, W. (1995). Fast effective rule induction. Proceedings of the Twelfth International Conference on Machine Learning, 115–123.
Cornelius, R. R. (1996). The science of emotion.Upper Saddle River, NJ: Prentice Hall.
Crystal, D. (2006). Language and the Internet (2nd ed.). Cambridge, UK: Cambridge University Press.
Das, S., & Chen, M. (2001). Yahoo! for Amazon: Extracting market sentiment from stock message boards. Proceedings of the Asia Pacific Finance Association Annual Conference (APFA), Bangkok, Thailand, July 22-25, Retrieved July 17, 2009 from: http://sentiment.technicalanalysis.org.uk/DaCh.pdf.
Denecke, K., & Nejdl, W. (2009). How valuable is medical social media data? Content analysis of the medical web. Information Sciences, 179(12), 1870-1880.
Derks, D., Bos, A. E. R., & von Grumbkow, J. (2008). Emoticons and online message interpretation. Social Science Computer Review, 26(3), 379-388.
Derks, D., Fischer, A. H., & Bos, A. E. R. (2008). The role of emotion in computer-mediated communication: A review. Computers in Human Behavior, 24(3), 766–785.
Diener, E., & Emmons, R. A. (1984). The independence of positive and negative affect. Journal of Personality and Social Psychology, 47(5), 1105-1117.
Ekman, P. (1992). An argument for basic emotions. Cognition and Emotion, 6(3/4), 169-200.
Esuli, A., & Sebastiani, F. (2006). SENTIWORDNET: A publicly available lexical resource for opinion mining. Proceedings of Language Resources and Evaluation (LREC) 2006, Retrieved July 28, 2009 from: http://tcc.fbk.eu/projects/ontotext/Publications/LREC2006-esuli-sebastiani.pdf.
Fox, E. (2008). Emotion science.Basingstoke: Palgrave Macmillan.
Fullwood, C., & Martino, O. I. (2007). Emoticons and impression formation. The Visual in Popular Culture, 19(7), 4-14.
Gamon, M. (2004). Sentiment classification on customer feedback data: noisy data, large feature vectors, and the role of linguistic analysis. Proceedings of the 20th international conference on Computational Linguistics, No.841.
Gamon, M., Aue, A., Corston-Oliver, S., & Ringger, E. (2005). Pulse: Mining customer opinions from free text (IDA 2005). Lecture Notes in Computer Science, 3646, 121-132.
Gill, A. J., Gergle, D., French, R. M., & Oberlander, J. (2008). Emotion rating from short blog texts. In Proceeding of the twenty-sixth annual SIGCHI conference on Human factors in computing systems (pp. 1121-1124). New York, NY: ACM.
Grinter, R. E., & Eldridge, M. (2003). Wan2tlk? everyday text messaging. CHI 2003, 441-448.
Hancock, J. T., Gee, K., Ciaccio, K., & Lin, J. M.-H. (2008). I'm sad you're sad: Emotional contagion in CMC. Proceedings of the ACM 2008 conference on Computer supported cooperative work, 295-298.
Hopkins, D. J., & King, G. (2010). A method of automated nonparametric content analysis for social science. American Journal of Political Science, 54(1), 229-247.
Huang, Y.-P., Goh, T., & Liew, C. L. (2007). Hunting suicide notes in web 2.0 - Preliminary findings. In Ninth Ieee International Symposium On Multimedia - Workshops, Proceedings (pp. 517-521). Los Alamitos: IEEE.
Huppert, F. A., & Whittington, J. E. (2003). Evidence for the independence of positive and negative well-being: Implications for quality of life assessment. British Journal of Health Psychology, 8(1), 107-122.
Kaji, N., & Kitsuregawa, M. (2007). Building lexicon for sentiment analysis from massive collection of HTML documents. In Proceedings of the 2007 Joint Conference on Empirical Methods in Natural Language Processing and Computational Natural Language Learning (pp. 1075-1083, retrieved July 1028 from: http://www.aclweb.org/anthology/D/D1007/D1007-1115.pdf).
Krippendorff, K. (2004). Content analysis: An introduction to its methodology.Thousand Oaks, CA: Sage.
Kukich, K. (1992). Techniques for automatically correcting words in text. ACM computing surveys, 24(4), 377-439.
Liu, H., Lieberman, H., & Selker, T. (2003). A model of textual affect sensing using real-world knowledge. Proceedings of the 2003 International Conference on Intelligent User Interfaces, IUI 2003, 125-132.
Mauss, I. B., & Robinson, M. D. (2009). Measures of emotion: A review. Cognition and Emotion, 23(2), 209-237.
Mishne, G. (2005). Experiments with mood classification in Blog posts. Style - the 1st Workshop on Stylistic Analysis Of Text For Information Access, at SIGIR 2005.
Mishne, G., & de Rijke, M. (2006). Capturing global mood levels using Blog posts. In Proceedings of the AAAI Spring Symposium on Computational Approaches to Analysing Weblogs (AAAI-CAAW) (pp. 145-152). Menlo Park, CA: AAAI Press.
Nardi, B. A. (2005). Beyond bandwidth: Dimensions of connection in interpersonal communication. Computer-Supported Cooperative Work, 14(1), 91-130.
Neviarouskaya, A., Prendinger, H., & Ishizuka, M. (2007). Textual affect sensing for sociable and expressive online communication. Lecture Notes in Computer Science, 4738, 218-229.
Ng, V., Dasgupta, S., & Arifin, S. M. N. (2006). Examining the role of linguistic knowledge sources in the automatic identification and classification of reviews. Proceedings of the COLING/ACL 2006 Main Conference, 611-618.
Pang, B., & Lee, L. (2004). Sentimental education: Sentiment analysis using subjectivity summarization based on minimum cuts. In Proceedings of ACL 2004 (pp. 271-278). New York: ACL Press.
Pang, B., & Lee, L. (2005). Seeing stars: Exploiting class relationships for sentiment categorization with respect to rating scales. Proceedings of the 43rd Annual Meeting of the ACL, 115-124.
Pang, B., & Lee, L. (2008). Opinion mining and sentiment analysis. Foundations and Trends in Information Retrieval, 1(1-2), 1-135.
Pennebaker, J., Mehl, M., & Niederhoffer, K. (2003). Psychological aspects of natural language use: Our words, our selves. Annual Review of Psychology, 54, 547-577.
Pennebaker, J. W., Mayne, T., & Francis, M. E. (1997). Linguistic predictors of adaptive bereavement. Journal of Personality and Social Psychology, 72(4), 863-871.
Pollock, J. J., & Zamora, A. (1984). Automatic spelling correction in scientific and scholarly text. Communications of the ACM, 27(4), 358-368.
Prabowo, R., & Thelwall, M. (2009). Sentiment analysis: A combined approach. Journal of Informetrics, 3(1), 143-157.
Read, J. (2005). Using emoticons to reduce dependency in machine learning techniques for sentiment classification. Proceedings of the ACL 2005 Student Research Workshop, 43-48.
Riloff, E., Patwardhan, S., & Wiebe, J. (2006). Feature subsumption for opinion analysis. Proceedings of the Conference on Empirical Methods in Natural Language Processing, 440-448.
Riloff, E., & Wiebe, J. (2003). Learning extraction patterns for subjective expressions. Proceedings of the 2003 Conference on Empirical Methods in Natural Language Processing (EMNLP-03), Retrieved April 11, 2010 from: http://www.cs.utah.edu/~riloff/pdfs/emnlp2003.pdf.
Russell, J. A. (1979). Affective space is bipolar. Journal of Personality and Social Psychology, 37(3), 345-356.
Schapire, R., & Singer, Y. (2000). BoosTexter: A boosting-based system for text categorization. Machine Learning, 39(2/3), 135-168.
Short, J. C., & Palmer, T. B. (2008). The application of DICTION to content analysis research in strategic management. Organizational Research Methods, 11(4), 727-752.
Snyder, B., & Barzilay, R. (2007). Multiple aspect ranking using the good grief algorithm. Proceedings of NAACL HLT.
Stone, P. J., Dunphy, D. C., Smith, M. S., & Ogilvie, D. M. (1966). The general inquirer: A computer approach to content analysis.Cambridge, MA: The MIT Press.
Stoppard, J. M., & Gunn Gruchy, C. D. (1993). Gender, context, and expression of positive emotion. Personality and Social Psychology Bulletin, 19(2), 143-150.
Strapparava, C., & Mihalcea, R. (2008). Learning to identify emotions in text, Proceedings of the 2008 ACM symposium on Applied computing (pp. 1556-1560). New York, NY: ACM.
Strapparava, C., & Valitutti, A. (2004). Wordnet-affect: an affective extension of wordnet. In Proceedings of the 4th International Conference on Language Resources and Evaluation (pp. 1083-1086). Lisbon.
Tang, H., Tan, S., & Cheng, X. (2009). A survey on sentiment detection of reviews. Expert Systems with Applications: An International Journal, 36(7), 10760-10773.
Thelwall, M. (2009). MySpace comments. Online Information Review, 33(1), 58-76.
Thelwall, M., Wilkinson, D., & Uppal, S. (2010). Data mining emotion in social network communication: Gender differences in MySpace. Journal of the American Society for Information Science and Technology, 21(1), 190-199.
Thurlow, C. (2003). Generation Txt? The sociolinguistics of young people's text-messaging. Discourse Analysis Online, 1(1), Retrieved January 3, 2008 from: http://extra.shu.ac.uk/daol/articles/v2001/n2001/a2003/thurlow2002003-paper.html.
Turney, P. D. (2002). Thumbs up or thumbs down? Semantic orientation applied to unsupervised classification of reviews. In: Proceedings of the 40th annual meeting of the Association for Computational Linguistics (ACL), July 6-12, 2002, Philadelphia, PA, 417-424.
Walther, J., & Parks, M. (2002). Cues filtered out, cues filtered in: computer-mediated communication and relationships. In M. Knapp, J. Daly & G. Miller (Eds.), The Handbook of Interpersonal Communication (3rd ed.) (pp. 529-563). Thousand Oaks, CA: Sage.
Watson, D. (1988). Intraindividual and interindividual analyses of positive and negative affect: their relation to health complaints, perceived stress, and daily activities. Journal of Personality and Social Psychology, 54(6), 1020-1030.
Watson, D., Clark, L. A., & Tellegen, A. (1988). Development and validation of brief measures of positive and negative affect: The PANAS scales. Journal of Personality and Social Psychology, 54(6), 1063-1070.
Wiebe, J., Wilson, T., Bruce, R., Bell, M., & Martin, M. (2004). Learning subjective language. Computational Linguistics, 30(3), 277-308.
Wiebe, J., Wilson, T., & Cardie, C. (2005). Annotating expressions of opinions and emotions in language. Language Resources and Evaluation, 39(2-3), 165-210.
Wilson, T. (2008). Fine-grained subjectivity and sentiment analysis: Recognizing the intensity, polarity, and attitudes of private states. University of Pittsburgh.
Wilson, T., Wiebe, J., & Hoffman, P. (2009). Recognizing contextual polarity: An exploration of features for phrase-level sentiment analysis. Computational linguistics, 35(3), 399-433.
Wilson, T., Wiebe, J., & Hwa, R. (2006). Recognizing strong and weak opinion clauses. Computational Intelligence, 22(2), 73-99.
Witten, I. H., & Frank, E. (2005). Data mining: Practical machine learning tools and techniques.San Francisco: Morgan Kaufmann.
Wu, C.-H., Chuang, Z.-J., & Lin, Y.-C. (2006). Emotion recognition from text using semantic labels and separable mixture models. ACM Transactions on Asian Language Information Processing, 5(2), 165-183.
[1] Thelwall, M., Buckley, K., Paltoglou, G., Cai, D., & Kappas, A. (2010). Sentiment strength detection in short informal text. Journal of the American Society for Information Science and Technology, 61(12), 2544–2558. Copyright © 2010 (American Society for Information Science and Technology)